A picture is worth a thousand words, but what if those words are faded or obscured? enter the world of ai image restoration techniques, where memories can be brought back to life and cherished once again.
Imagine having the power to turn back time and restore your precious photos to their original glory. thanks to artificial intelligence, this is no longer a figment of our imagination but a reality we can all enjoy.
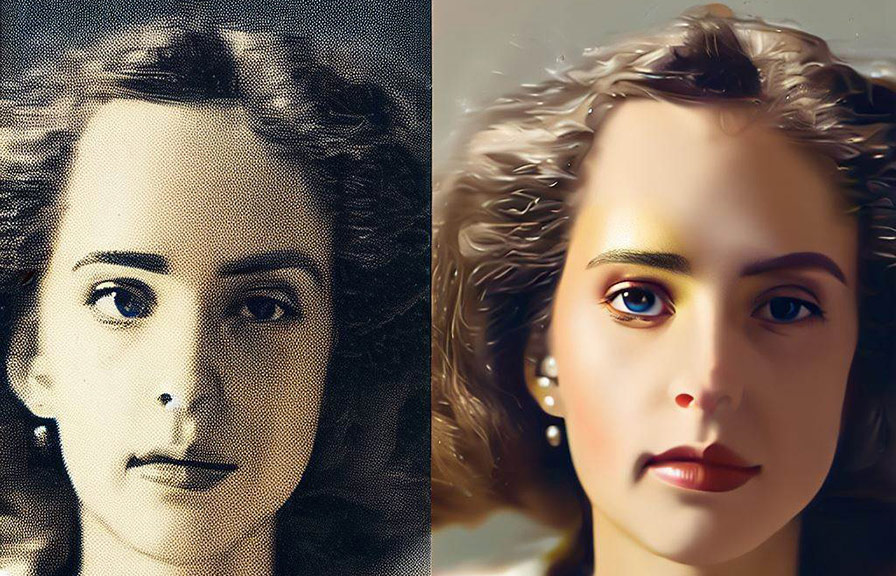
The power of ai in restoring images
The rise of ai in recent years has led to groundbreaking advancements in various fields, and image restoration is no exception. ai-based image restoration techniques have the potential to recover lost details, remove noise, and even colorize black-and-white photos.
Did you know that ai image restoration techniques can also help in criminal investigations? by enhancing low-quality or damaged images, investigators can obtain vital information and evidence to solve cases. the power of ai in restoring images extends from our personal photo albums to matters of public safety.
How ai image restoration techniques work
To understand how ai image restoration techniques work, it's crucial to dive into the two main approaches: supervised learning and unsupervised learning.
Supervised learning
In supervised learning, the ai model is trained using a large dataset of paired images – one set of degraded images and another set of their high-quality counterparts. the ai model learns to restore images by comparing and minimizing the differences between its output and the high-quality images.
Once trained, the ai model can be applied to new degraded images to restore them.
One popular supervised learning method is the convolutional neural network (cnn). cnns are designed to automatically and adaptively learn spatial hierarchies of features from the input images. they have shown remarkable success in image restoration tasks, such as denoising, deblurring, and super-resolution.
Unsupervised learning
Unsupervised learning, on the other hand, doesn't require paired datasets. instead, the ai model learns to restore images by extracting features and patterns from the input images themselves. this approach is particularly useful when high-quality counterparts are not available or too costly to obtain.
Generative adversarial networks (gans) are a popular unsupervised learning technique for image restoration. gans consist of two neural networks, a generator and a discriminator, that compete against each other. the generator creates restored images, while the discriminator evaluates their quality.
Through this adversarial process, both networks learn and improve, resulting in high-quality image restoration.
Examples of ai image restoration techniques
Various ai image restoration techniques have been developed, each with its own strengths and applications. here are some notable examples:
1. deep image prior
Deep image prior is an unsupervised image restoration method that leverages the structure of cnns to restore images. instead of being trained on a dataset, the cnn learns directly from the degraded image. the output is then fine-tuned to restore the image without overfitting to the noise or artifacts.
This method has been successful in tasks such as denoising, inpainting, and super-resolution.
2. esrgan: enhanced super-resolution generative adversarial networks
Esrgan is a gan-based method designed for single-image super-resolution. it improves upon the original super-resolution gan (srgan) by introducing a new architecture and loss function. esrgan has demonstrated impressive results in increasing image resolution while maintaining fine details, textures, and sharpness.
3. deoldify
Deoldify is a gan-based colorization method that brings new life to black-and-white photos by adding realistic and vibrant colors. trained on a large dataset of historical images, deoldify can predict and apply appropriate colors to a variety of subjects, including portraits, landscapes, and urban scenes.
Challenges and future directions
While ai image restoration techniques have made significant strides, there are still challenges to overcome. for instance, selecting the appropriate method for a specific restoration task remains a complex decision. additionally, overfitting and artifacts can occur, leading to unnatural or distorted results.
However, as ai continues to evolve, so too will image restoration techniques. as advancements are made in ai algorithms, hardware, and training methodologies, we can expect even higher-quality image restoration in the future.
Conclusion
Ai image restoration techniques offer a remarkable opportunity to restore and preserve our precious memories. from personal photo albums to criminal investigations, these techniques have the potential to make a significant impact on our lives.
As ai continues to progress, the possibilities for image restoration are limitless, breathing new life into our most cherished moments.